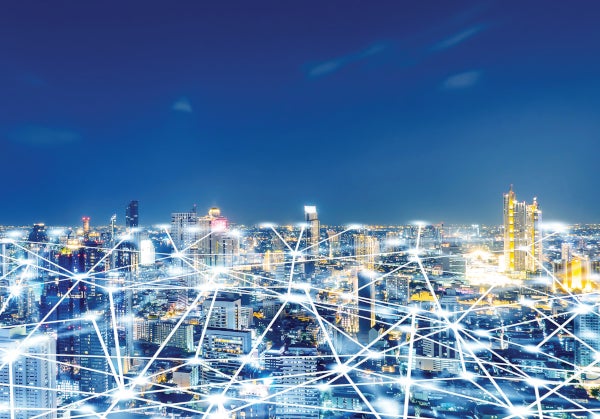
A few years back, the term ‘artificial intelligence’ may have carried a vaguely threatening, dystopian quality. With machine learning technologies on the cusp of going mainstream, you didn’t have to go far to encounter gloomy predictions about robot takeovers and mass job losses.
A 2015 report by the Lords’ Select Committee on Digital Skills estimated that over a third of UK jobs were at risk of being automated over the next two decades. Two years later, a report by McKinsey estimated that some 400–800 million people could be displaced by automation globally – and forced to find new jobs by 2030.
Strikingly, many of these people could be white-collar professionals, their roles imperilled by machines just like factory or agricultural workers before them. According to a joint 2015 study by the University of Oxford and Deloitte, the most vulnerable individuals will be those performing back office or administrative functions. The study’s ‘calculator’ estimated that accountants have a 95% chance of losing their jobs – rising to 97% for bookkeepers and payroll managers.
Robot takeover?
These predictions haven’t been revised exactly – in fact, the pandemic has conspicuously accelerated the trend towards automation. Even so, our growing familiarity with AI is giving rise to a more encouraging counter-narrative. Simply put, while many jobs may indeed be displaced, a wave of new roles will emerge in their stead. “Any job losses from automation are likely to be broadly offset in the long run by new jobs created as a result of the larger and wealthier economy made possible by these new technologies,” claimed the authors of a PwC AI study, aptly titled ‘Will robots really steal all our jobs?’.
Meanwhile, professionals across every sector are finding that AI technologies are actually changing their jobs for the better. By automating the mundane tasks they never loved in the first place, AI has freed them up to focus on more creative or strategic pursuits – areas where the human brain has an edge over any machine.
Nowhere is this clearer than in the treasury function – where many repetitive manual tasks are already being performed by AI. You might, of course, think that treasury jobs would be at risk, given the similarities with accountancy. Yet despite the ways automation is changing the sector, serious job losses have yet to materialise.
“The threat does seem to be overstated in the treasury function,” explains Sarah Boyce, associate director, policy and technical, at the Association of Corporate Treasurers (ACT). “Treasury remains an area requiring a combination of deep technical expertise and the ability to communicate complex issues with internal and external stakeholder – areas which automation are unlikely to replace.”
In fact, the Oxford/Deloitte study noted that jobs requiring a high degree of social intelligence and negotiating skills were considerably less at risk from machines. The same applies to roles demanding original thinking, or forcing employees to think on their feet.
Boyce adds that treasury teams are generally small with little slack – meaning there simply isn’t scope for slashing headcounts. Rather, the introduction of AI helps teams shift their focus away from routine matters and towards more strategic tasks.
“This enables them to add greater value to the business as, for example, they spend less time on identifying cash requirements and more time on effective funding of the business,” Boyce continues. “It also improves the controls environment, as there is less opportunity for human error.”

Changing functions
This is not to say the shift towards AI hasn’t been profound. The introduction of Robot Process Automation (RPA), which began to hit the financial mainstream around 2015, has enabled treasurers to automate many of the tasks at the lower end of the value chain.
According to Gartner, 80% of finance leaders have implemented, or are planning to implement, RPA. This trend has become firmly entrenched over the past few years, as the role of the corporate treasurer has become more challenging. Faced with a slew of regulatory changes, and the pressures of the pandemic, overworked treasurers have been doing all they can to improve efficiency.
Simply put, RPA refers to a form of automation software whereby the user defines a set of instructions for a bot to perform. You give the robot some logical rules, and the rest is down to the machine – it will perform the given task unflaggingly, inexpensively, and without making mistakes.
To date, RPA’s main applications in treasury have been in the cash management and payment space: cash pooling, data consolidation, invoicing, auditing and so forth. The upshot is that these processes are becoming far more efficient and accurate. Research from EY suggests that RPA can help teams regain up to 70% of the time they would have spent on manual data processes.
Increasingly, RPA may be used across more complex functions too. It isn’t quite the same as AI – unlike a deep neural network, it is confined to a particular function and doesn’t learn as it goes. As soon as you want to do something outside of its strict parameters, a human will need to get involved.
Even so, this distinction may soon be elided as RPA tools start to be used in conjunction with AI. Over time, we may see these technologies being used to automate entire workflows.
45%
The percentage of repetitive tasks now automated or augmented with AI, robotics and RPA.
IDC
“Some of the roadblocks include lack of understanding of the benefits; lack of understanding to ask the right questions; identifying exactly how AI can add value to the process; and lack of resources or budget to implement new solutions and processes.”
According to a 2021 report by the research and advisory company Forrester: “Customers want to scale existing bot environments and extend the scope of their automation projects beyond classic desktop-based tasks to more complex processes.”
Once machine learning is used more broadly, it could pave the way for a wholesale transformation of the treasury function. Possibilities include better fraud detection, improved pattern recognition, and automated financial risk assessments.
A machine learning powered system can also make sophisticated forecasts and draw from huge data sources – including ‘unstructured data’ like social media – to make unassisted decisions. However, the real draw for many treasurers will be having more real-time information at their disposal, so as to improve their own decision-making. In this scenario, the treasurer is performing a strategic, insightful role, albeit assisted by automation.
50%
The percentage of workers who will require some upskilling or reskilling in the next five years.
World Economic Forum’s Future of Jobs Report, 2020
To date, AI-powered treasury bots have been used in areas like liquidity planning and FX exposure planning. They are also proving useful in cash forecasting, and are particularly good at uncovering discrepancies that might otherwise go undetected. For instance, PNC Treasury Management recently announced a new cash management application that uses AI and machine learning to produce cash forecasts based on historical data.
“Removing complexities and obstacles for ongoing tasks is central to our work, and we know PINACLE Cash Forecasting will be a critical resource for our clients as they continue to operate in disrupted working environments” said Chris Ward, executive vice president for PNC Treasury Management, in a press release.
Roadblocks ahead
Even so, fully embracing AI is not yet at the top of the priority list for many companies – while many applications remain at a pilot stage. Machine-learning technologies are generally more complex to implement than RPA. They’re also less well-tested, meaning treasurers – a risk-averse bunch by profession – may be reluctant to take the leap.
“Investment in Machine Learning remains comparatively low,” says Boyce. “The most popular focus for ML is on cash forecasting and its application to better understand and predict customer sales – especially in sectors such as retail and hospitality.”
She adds that AI-based tools are merely enablers to address particular challenges – they do not automatically suit everyone and many companies will have barriers to implementation. “Some of the roadblocks include lack of understanding of the benefits; lack of understanding to ask the right questions; identifying exactly how AI can add value to the process; and lack of resources or budget to implement new solutions and processes.”
Another stumbling block might be regulatory concerns. The frameworks around AI are still evolving, and there are questions around accountability and bias that aren’t necessarily easy to resolve.
So is now the time to invest in AI? According to many commentators of a futurist bent, that is indeed the case – failing to take the leap will ensure you’re left behind. Yet in practical terms, a certain level of caginess is to be expected, as we are dealing with many unknowns.
What seems clear, at any rate, is that treasury teams will spend less time than ever on back-office tasks and more on the meaningful, problem-solving aspects of their role. Corporate treasurers may not lose their jobs – but they will need to become more flexible as their technological ecosystem changes. In the meantime, Boyce advises taking a cautious and measured approach to automation.
“It’s important to get the basics right and to standardise existing processes before embarking on any automation programme,” she says. “There is still a lot of development going on in this space and it’s best to wait and see how these settle down into a mature and reliable solution for what remains a business critical function.”